Ecology
Learn to analyse Ecological data through Galaxy.
Requirements
Before diving into this topic, we recommend you to have a look at:
Material
You can view the tutorial materials in different languages by clicking the dropdown icon next to the slides (slides) and tutorial (tutorial) buttons below.Data and Metadata Management
These tutorials are focusing on data and metadata management in Ecology.
Data access
These lessons focus on ways to access data classicaly used in Ecology
Lesson | Slides | Hands-on | Recordings | Input dataset | Workflows |
---|---|---|---|---|---|
QGIS Web Feature Services |
Data preprocessing
These lessons focus on manners to preprocess data used in Ecology
Lesson | Slides | Hands-on | Recordings | Input dataset | Workflows |
---|---|---|---|---|---|
Biodiversity data exploration | |||||
Checking expected species and contamination in bacterial isolate | |||||
Cleaning GBIF data for the use in Ecology |
Data analysis
These lessons focus on ways to analyse data in Ecology
Data visualization
These tutorials showcase data visualization in Ecology
Lesson | Slides | Hands-on | Recordings | Input dataset | Workflows |
---|---|---|---|---|---|
Visualization of Climate Data using NetCDF xarray Map Plotting | |||||
Visualize EBV cube data with Panoply netCDF viewer |
Frequently Asked Questions
Common questions regarding this topic have been collected on a dedicated FAQ page . Common questions related to specific tutorials can be accessed from the tutorials themselves.
Follow topic updates rss-feed with our RSS Feed
Community Resources
Community Home Maintainer HomeEditorial Board
This material is reviewed by our Editorial Board:

Contributors
This material was contributed to by:


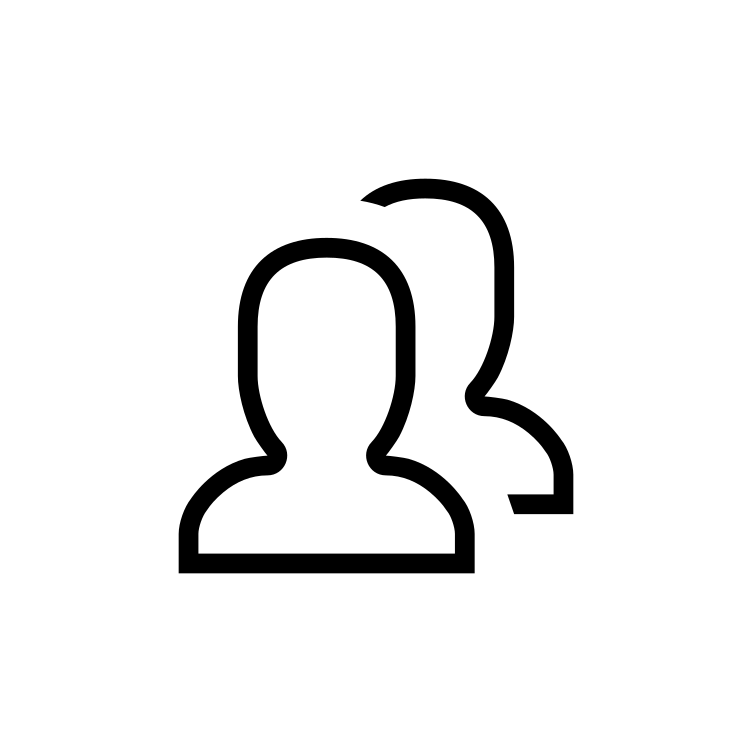
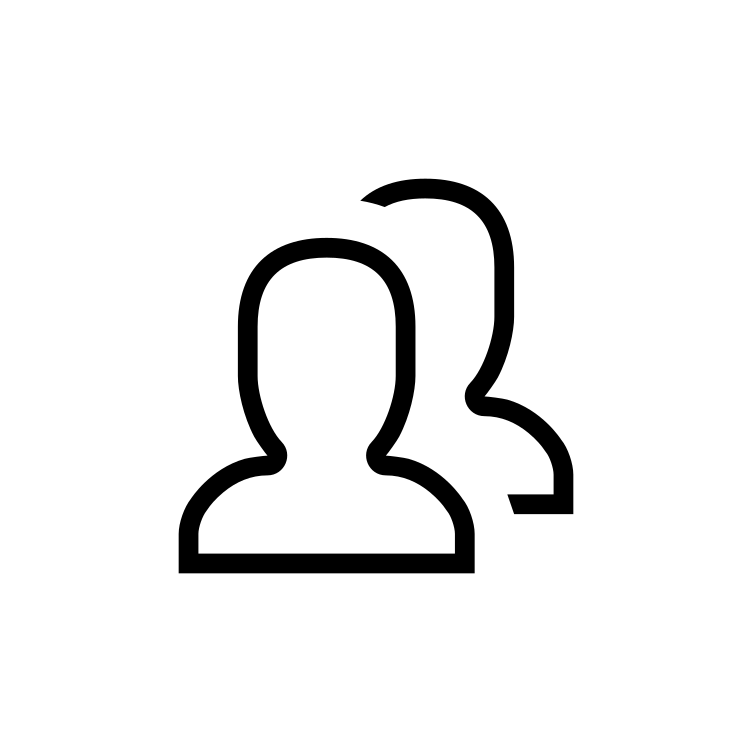












Funding
These individuals or organisations provided funding support for the development of this resource
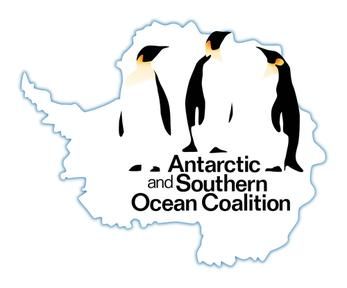
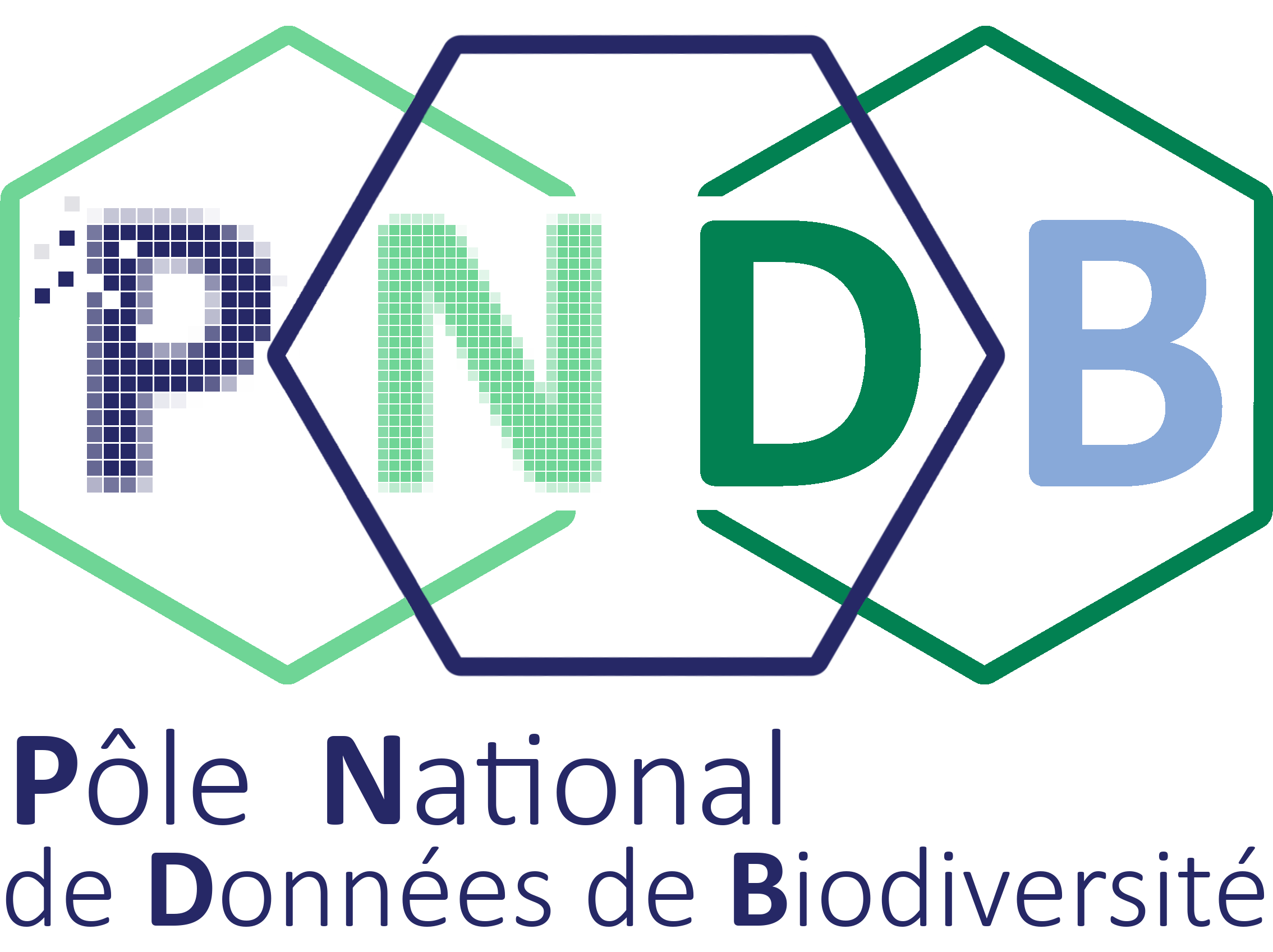


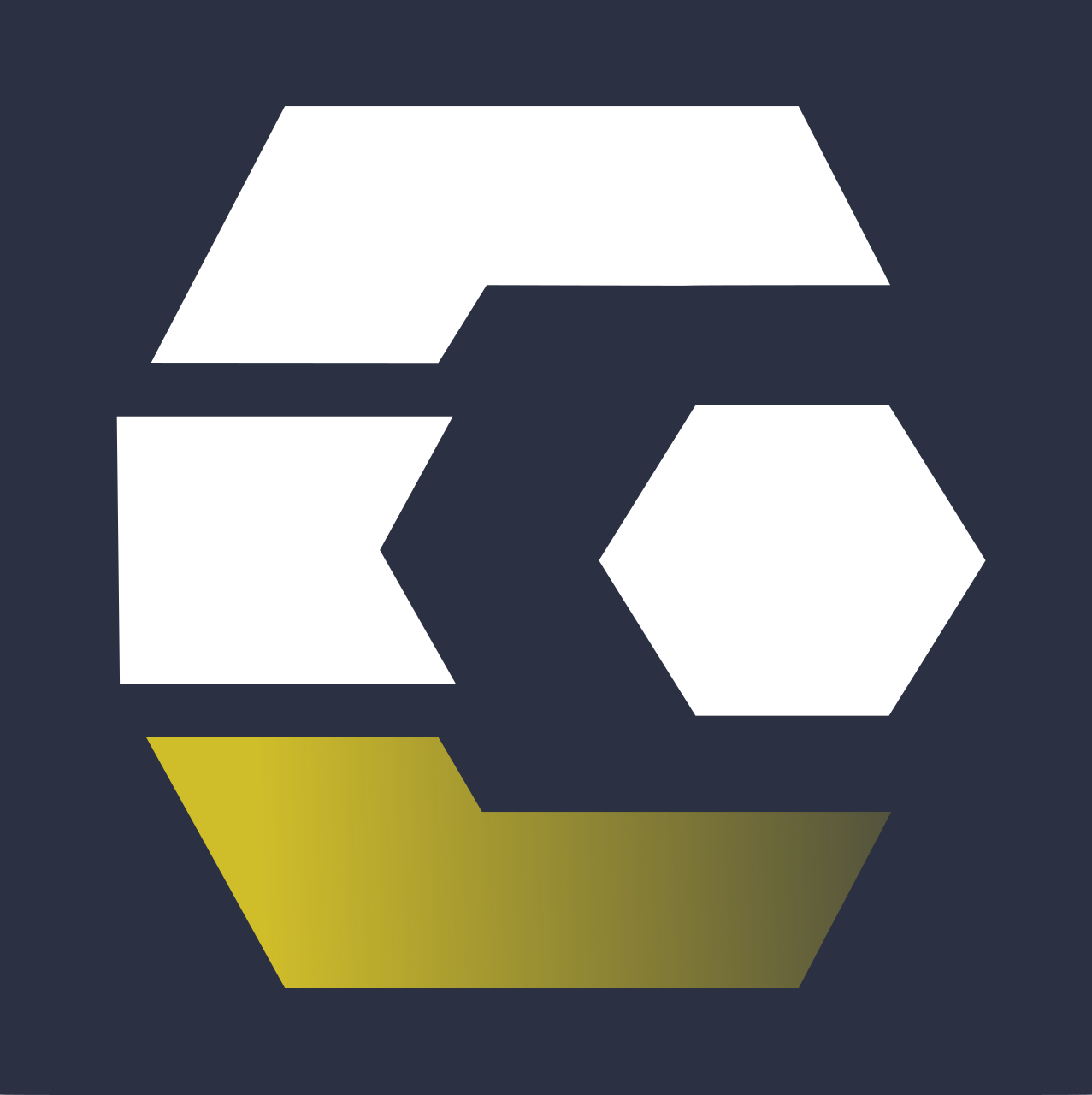
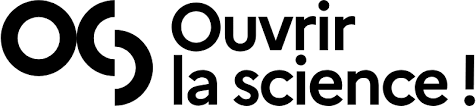
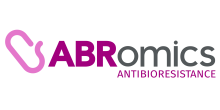
References
-
Reto Schmucki et al: A regionally informed abundance index for supporting integrative analyses across butterfly monitoring schemes
The rapid expansion of systematic monitoring schemes necessitates robust methods to reliably assess species' status and trends. Insect monitoring poses a challenge where there are strong seasonal patterns, requiring repeated counts to reliably assess abundance. Butterfly monitoring schemes (BMSs) operate in an increasing number of countries with broadly the same methodology, yet they differ in their observation frequency and in the methods used to compute annual abundance indices. Using simulated and observed data, we performed an extensive comparison of two approaches used to derive abundance indices from count data collected via BMS, under a range of sampling frequencies. Linear interpolation is most commonly used to estimate abundance indices from seasonal count series. A second method, hereafter the regional generalized additive model (GAM), fits a GAM to repeated counts within sites across a climatic region. For the two methods, we estimated bias in abundance indices and the statistical power for detecting trends, given different proportions of missing counts. We also compared the accuracy of trend estimates using systematically degraded observed counts of the Gatekeeper Pyronia tithonus (Linnaeus 1767). The regional GAM method generally outperforms the linear interpolation method. When the proportion of missing counts increased beyond 50%, indices derived via the linear interpolation method showed substantially higher estimation error as well as clear biases, in comparison to the regional GAM method. The regional GAM method also showed higher power to detect trends when the proportion of missing counts was substantial. Synthesis and applications. Monitoring offers invaluable data to support conservation policy and management, but requires robust analysis approaches and guidance for new and expanding schemes. Based on our findings, we recommend the regional generalized additive model approach when conducting integrative analyses across schemes, or when analysing scheme data with reduced sampling efforts. This method enables existing schemes to be expanded or new schemes to be developed with reduced within‐year sampling frequency, as well as affording options to adapt protocols to more efficiently assess species status and trends across large geographical scales. -
Vitor H. F. Gomes et al: Species Distribution Modelling: Contrasting presence-only models with plot abundance data
Species distribution models (SDMs) are widely used in ecology and conservation. Presence-only SDMs such as MaxEnt frequently use natural history collections (NHCs) as occurrence data, given their huge numbers and accessibility. NHCs are often spatially biased which may generate inaccuracies in SDMs. Here, we test how the distribution of NHCs and MaxEnt predictions relates to a spatial abundance model using inverse distance weighting (IDW)