Gallantries Grant - Intellectual Output 4 - Data analysis and modelling for evidence and hypothesis generation and knowledge discovery
purlPURL: https://gxy.io/GTN:P00015Comment: What is a Learning Pathway?
We recommend you follow the tutorials in the order presented on this page. They have been selected to fit together and build up your knowledge step by step. If a lesson has both slides and a tutorial, we recommend you start with the slides, then proceed with the tutorial.
This Learning Pathway collects the results of Intellectual Output 4 in the Gallantries Project
Success Criteria:
- SC4.1) Statistical analysis. This will build on the basic statistics covered in IO1 to give a much better statistical comprehension often needed in more advanced analyses like modeling.
- SC4.2) Interactive data visualisation. For most cases, existing visualisations are sufficient, but knowing which visualisation is appropriate and why can be a key point often missed. Additionally sometimes analyses will require custom visualisation such as for geographic information system data.
- SC4.3) Hypothesis generation. When a researcher is handed a large pile of data, figuring out which questions to ask, and what the expected answer is, is the first step of good science.
- SC4.4) Advanced data modelling. Given a hypothesis for some data, a researcher should know how to model changes across some unknown variables, predicting into the future or filling in potential missing gaps in data.
Year 1: Biodiversity data handling and visualisation
learners will understand how to handle biodiversity data and analyse it, as well as elements of visualisation, identifying the optimal visualisation for a dataset. [SC1.1,SC1.4, SC2.1, SC2.3, SC4.1-3]
Lesson | Slides | Hands-on | Recordings |
---|---|---|---|
Compute and analyze biodiversity metrics with PAMPA toolsuite | |||
Regional GAM | |||
Biodiversity data exploration | |||
Cleaning GBIF data for the use in Ecology |
Year 2: Metabarcoding and environmental DNA data analysis
analysis of environmental DNA samples requires integrative analysis of highly diversified samples, and new techniques to scale with the data [SC1.4, SC1.5, SC2.1, SC3.1, SC4.1-4]
Lesson | Slides | Hands-on | Recordings |
---|---|---|---|
Metabarcoding/eDNA through Obitools |
Year 3: Species distribution modeling
As an application of data modeling, we will use species migration and biodiversity to teach learners how to build models for complex data and visualise the results. [SC1.1, SC2.4, SC4.1-4]
Lesson | Slides | Hands-on | Recordings |
---|---|---|---|
Species distribution modeling |
Editorial Board
This material is reviewed by our Editorial Board:


Funders
This material was funded by:
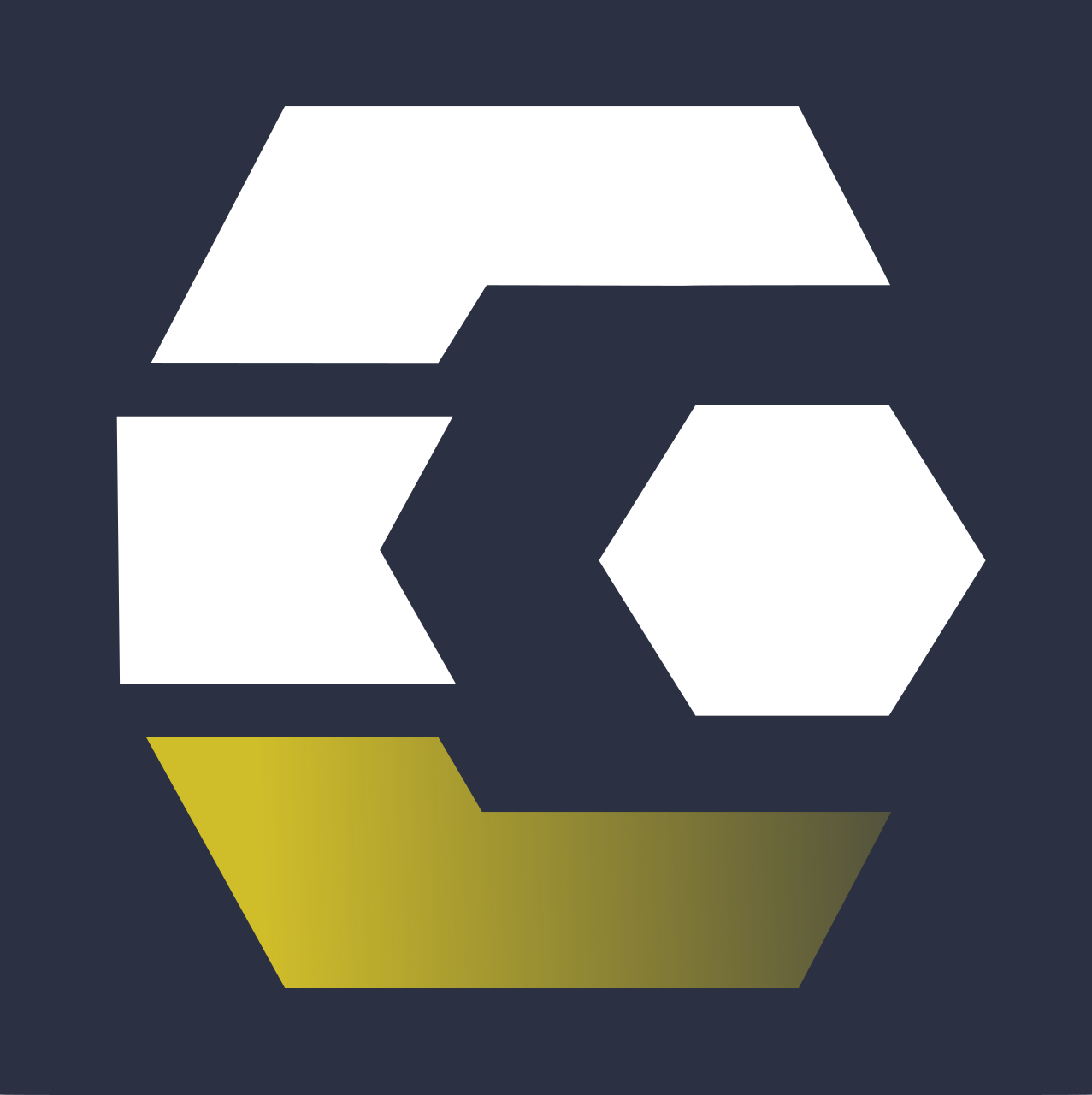